AI Technology
Solution for smarts sensors and cognitive storageTrainable lifelong AI
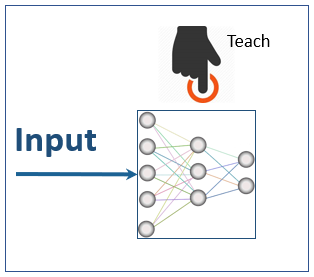
In-situ learning, auto-correction, no duplication
Learning an new example can occur anytime and the NeuroMem neurons can do without supervision.
Because you cannot learn what you already know, they first attempt to recognize the sample, and subsequently decide to commit a new neuron if novelty, adjust the weight of existing neurons if contradiction, or do nothing. This entire operation requires a communication between all the neurons, but only takes a few microseconds thanks to a Winner-Takes-All parallel logic.
Neurons can also be pre-trained by loading a knowledge file previously built by a NeuroMem network.
Responsible AI
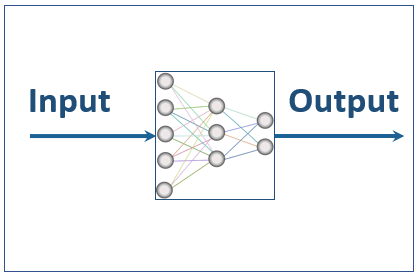
Get real values, not just probabilities
Because the closest match can still be far, the NeuroMem neurons output additional information and do not hesitate to report when they do not know or are unsure about a classification.
Such behavior is much preferred than probabilities when there is cost of a mistake. Acknowledgment of uncertainties enriches the decision process and can suggesting more training or the recourse to a second opinion such as another network trained with a different feature. For the best robustness, the final decision may require minimum consensus between neurons of a same or multiple networks.
Explainable AI
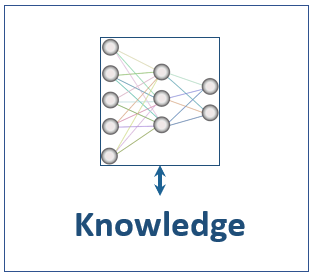
Not a black box, but a knowledge base
When neurons learn a new pattern, the decision is actually made collectively. If they agree to learn a novel pattern, it is actually stored in the memory of the next available neuron along with its category and a weight established by the neural network.
Consequently, the content of the neurons not only represent a knowledge, but is also the log of all the patterns that have been retained as novelty at the time they were taught.
2 modes of classification
Radial Basis Function (RBF)
- Highly adaptive model generator
- Non linear classifier
- Report uncertainties – for robust decision making
- Report novelties – for prediction and learning causality
K-Nearest Neighbor (KNN)
- Closest match in msec regardless of the number of models
- Ultra-fast patented Winner-Takes-All for the Search and Sort
- Commonly used for sorting and clustering algorithms
Scalable architecture
Design your solution with provisions to expand as your knowledge grows.
- Homogenous assembly of identical neuron cells with no supervisor
- Neuron cell = memory + processing logic
- Full interconnect between neuron cells
- Deployable as a software IP or a bank of chips
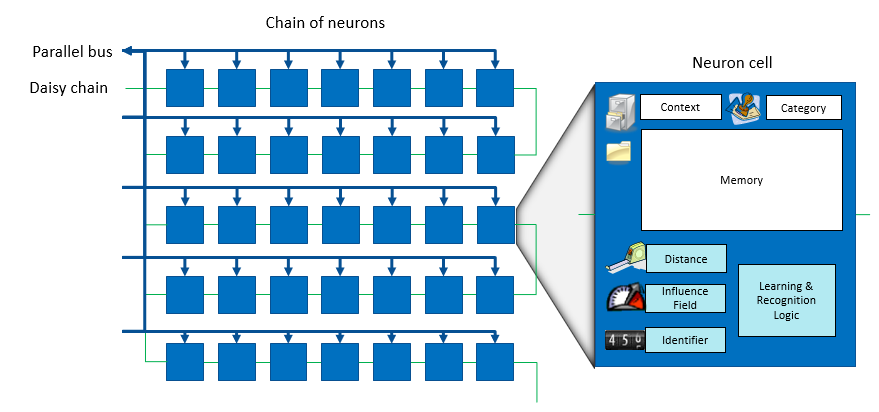
- How do the neurons learn?
- How does a NeuroMem network model a decision space?
- When to use KNN versus RBF?
- What are the outputs of a NeuroMem network?
- How to train a NeuroMem network with multiple features