Reference designs
NeuroMem-poweredNeuroMem for Smart Sensors
Power your sensors with edge intelligence. Whether on an industrial machinery, under the hood of a vehicle, or around a wrist, the ability to learn and classify patterns coming directly from a sensor is game-changing.
NeuroMem for Smart Storage
Turn your storage devices into local, secure and configurable engines to analyze your data. Whether it is text, audio or image data, comprehend content or detect novelty without moving your data.
Learning and recognition at the edge
- Train on your hardware
- Option to load a knowledge previously exported from a NeuroMem engine
- 100K+ vector recognition/sec
- Deterministic latencies
- Low power consumption
- Scalable network capacity
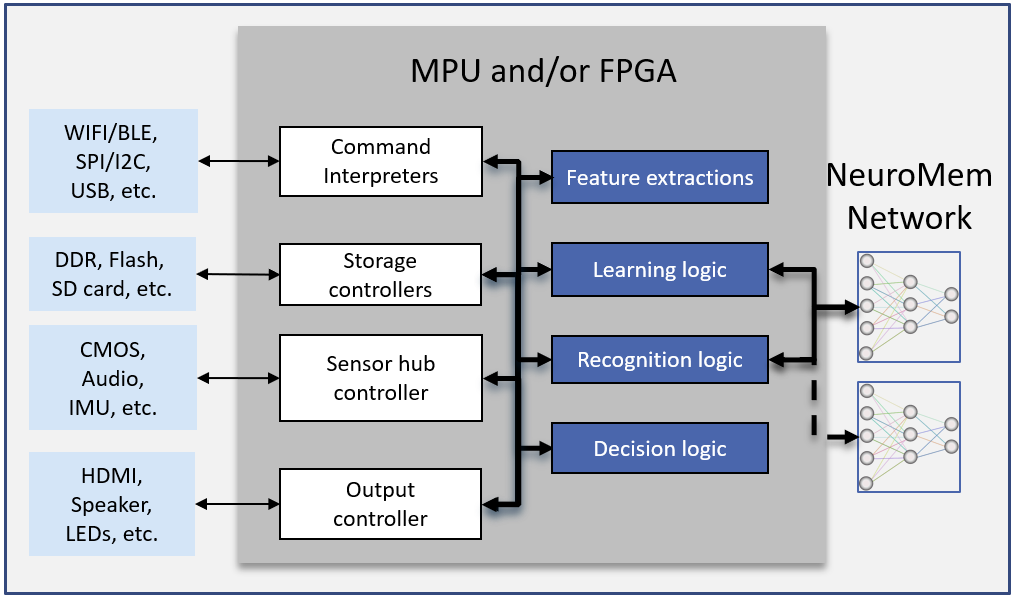
A typical NeuroMem-powered platform is composed of a processing unit (MCU, FPGA or both) interfaced to a bank of NeuroMem chips through the NeuroMem parallel bus or an intermediary serial communication controller. Depending on the targeted application, GPIOs and communication ports are connected to the MCU or FPGA. A Flash memory or SD card are convenient to store locally the MCU/FPGA configuration files and the NeuroMem pre-trained knowledge files if any.
The NeuroMem parallel architecture enables a seamless scalability of the network by cascading chips.
- Interested in one of the following reference designs?
- Want the same hardware using a different NeuroMem chip?
- Need customization for your project?
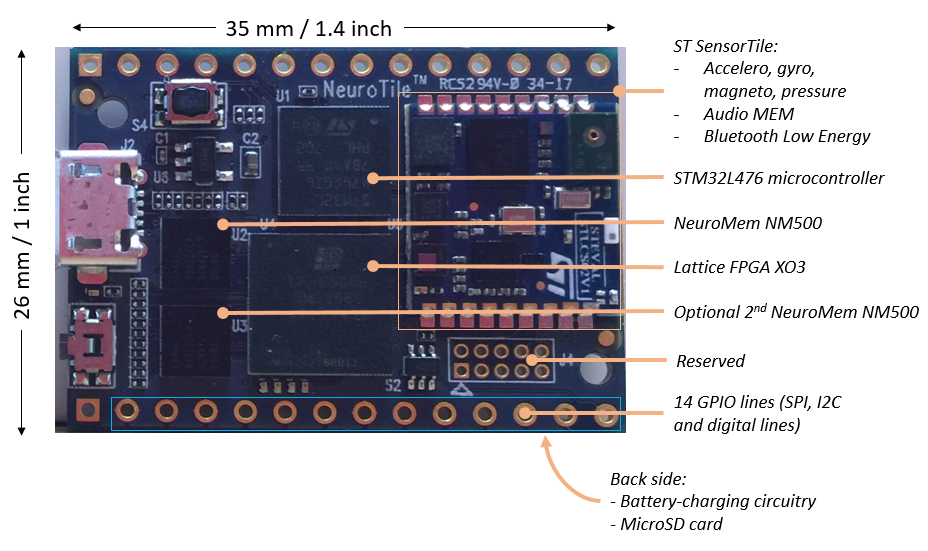
NeuroTile
Smallest sensory hub capable of real time machine learning. Based on a STM32 microcontroller and a NeuroMem neural network, it can monitor signals coming from its 3D accelerometer and gyro, a magnetometer and microphone. You can configure the learning and recognition engine from a file stored on its micro SD card or through a Blue Tooth communication. NeuroTile is delivered with a library compatible with the Arduino IDE and Eclipse.
NeuroStamp
Powerful learning and inference engine with the size of a stamp and ready to learn and recognize patterns from images, time series, and other data. It features a bank of 4032 neurons (7 NM500 chips) consuming less energy and with less complexity than microprocessors. The neurons are accessed through simple SPI protocol, but additional communication and processing logic can be loaded in the FPGA.
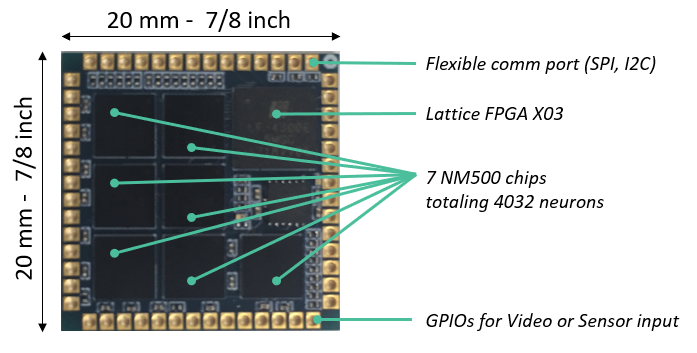
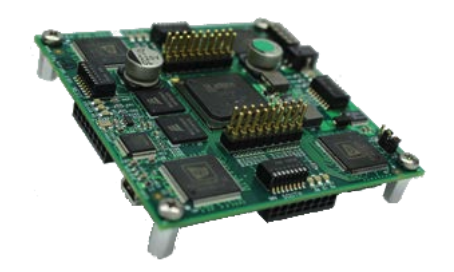
NeuroStack
Learn and classify patterns free of worries about the sizing of your neural network. Start with a single master USB board populated with 4096 neurons and stack more slave boards as you need more neurons.