by Guy Paillet | Nov 10, 2020 | Corporate News |
Moving Picture, Audio and Data Coding by Artificial Intelligence (MPAI) is born with the mission of taking advantage of Artificial Intelligence to define data coding standards. The NeuroMem neurons are perfect candidates to develop these standards with use models including non supervised annotation, novelty detection and classification without duplication, data modeling and compression.
The MPAI community is no less than the successor of MPEG and founded by the same Leonardo Chiariglione. Guy Paillet, president of General Vision, is honored to have been invited by Mr. Chiariglione to join MPAI as a board member and Vice President.
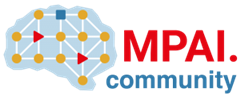
MPAI Community
Stay tune to hear more about the process of the community.
by Guy Paillet | Jul 4, 2020 | Product News |
Whether you are interested in video, sounds, vibrations or other sensors, you can teach NeuroMem networks with examples in real time and start immediately monitoring their response (or lack of response in the case of novelty detection)
With the new NeuroShield HDK for the ZYNQ7000 SOC, developers can easily integrate real-time pattern learning and recognition into their applications using the software programmability of an ARM®-based processor and/or the hardware programmability of an FPGA.
The pairing of the ZYNQ and NeuroShield grants the ability to surround a powerful processor with a unique digital neural network, tailored for whatever AI application is being conquered. Video, audio, vibration and other sensory inputs can be acquired, formatted, learned and immediately recognized. Decision can be taken on-board to control an actuator, transmit or store data of interest. Typical applications include detection of abnormal vibrations in an appliance, classification of outdoor noises from glass debris to bird songs, monitoring of a flame (or absence of a flame), identification of a person or object in a field of view, etc.
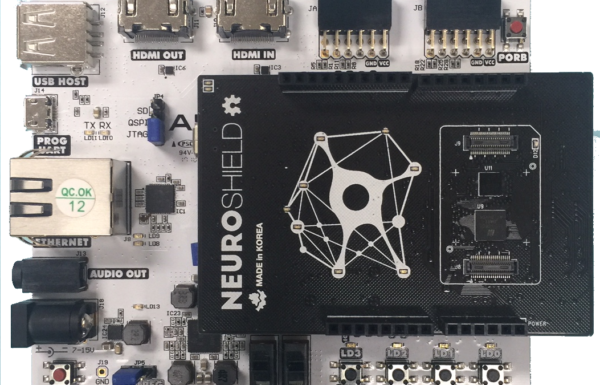
The NeuroShield board features a digital neural network of 576 neurons, expandable to 4032 neurons. It can learn features extracted from single or multiple sensors upon the push of a button or other external or programmatic events managed by the ARM or FPGA of the ZYNQ SOC. As soon as a learning has occurred, the neurons can be queried and depending on the application, the ARM or FPGA can retrieve a simple recognition status (Identified, Uncertain or Unknown), the category of the closest match or a detailed classification integrating the response of all firing neurons.
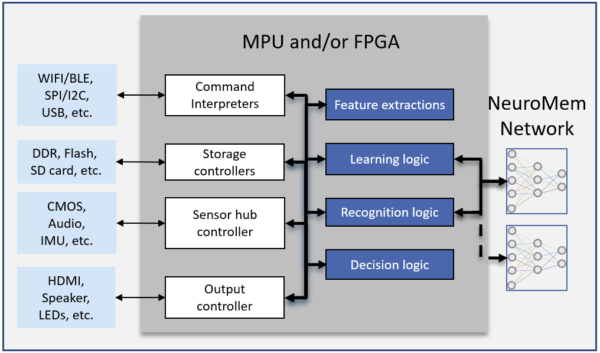
Unlike a conventional CNN or DL approach, the NeuroMem neurons are capable of intrinsic learning and recognition. They learn autonomously and collectively on the NeuroShield board and adapt their knowledge in real-time so new examples are taken into account at the next recognition. Learning does not require access to massive databases of annotated models and the teacher can observe the accuracy and throughput of the network after teaching a few relevant examples of each category. Note that the training can also be done offline since the NeuroShield can also be interfaced to a PC via its USB connector. In either case, the knowledge built by the neurons can be saved and ported between platforms using General Vision’s tools.
Currently supported platforms:
- Digilent Arty Z7 (switches & leds, Gige, HDMI, audio, pmods, SD card, etc)
- Avnet MiniZed (switches & leds, wifi, pmods, etc)
- HDK comes with Vivado and SDK projects and can be easily updated to support other ZYNQ7000 development boards
by Guy Paillet | Feb 10, 2020 | Customer success, Home page News |
NeuroTechnologijos installs a NeuroMem-powered monitoring system in a steel blaster furnace in Magnitogorsk – Russia. Its solution is composed of a bank of NT Adaptive Controllers designed and manufactured by NeuroTechnologijos and mounted in an industrial enclosure. Each NT Adaptive Controller receives signals from the machinery equipment and uses a NeuroMem neural network to verify that the signals stay within normal waveforms in amplitude, frequency and envelope. If novelties are detected, a second neural network can automatically learn the new waveforms for later review by a human supervisor.
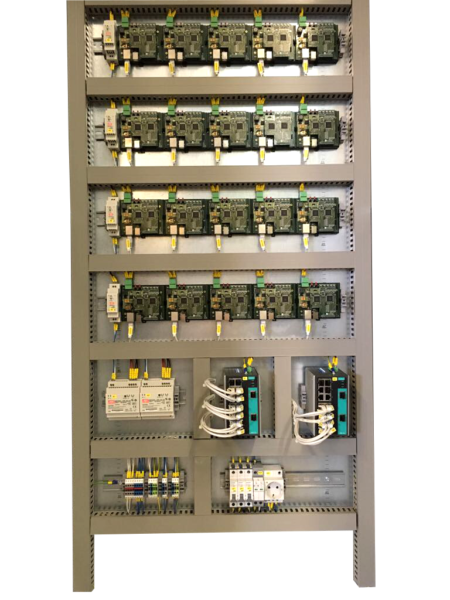
by Guy Paillet | Dec 1, 2018 | Corporate News |
General Vision has developped quintessential applications such as CogniSight engines, IntelliGlass and Cognitive SSD for practical AI solutions. Read more >>